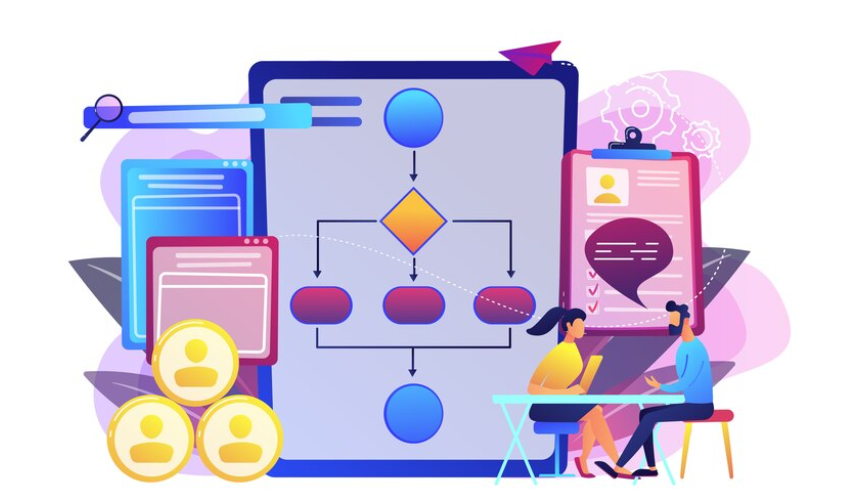
Introduction
Federated Learning (FL) stands at the forefront of a technological revolution, offering a novel approach to machine learning that challenges the conventional centralized model. In this article, we unravel the intricacies of Federated Learning, exploring its core principles, applications, and the transformative impact it holds for the future of collaborative machine learning without the need for centralized data storage.
You should also visit trendzguruji.me computer to get more information.
Demystifying Federated Learning
Understanding Federated Learning
Federated Learning is a machine learning paradigm that enables model training across decentralized edge devices or servers holding local data samples, without exchanging them. This section provides an in-depth understanding of how FL operates, emphasizing the collaborative nature that sets it apart from traditional centralized learning approaches.
The Rise of Collaborative Intelligence
Explore the evolution of collaborative intelligence and its role in shaping the landscape of machine learning. Discuss how Federated Learning addresses privacy concerns by keeping data localized and enabling multiple entities to contribute to the development of a global model without sharing sensitive information.
How Federated Learning Works
Decentralized Model Training
Dive into the mechanics of Federated Learning, elucidating the process of model training across distributed devices. Discuss the role of federated optimization algorithms and how they enable the global model to learn from local data without compromising privacy.
Federated Averaging
Explore the widely-used Federated Averaging algorithm, shedding light on how it aggregates locally trained models from various devices to create a more robust and accurate global model. Discuss the iterative nature of this process and its significance in achieving convergence.
Applications of Federated Learning
Personalized Recommendations
Examine how Federated Learning is reshaping recommendation systems, allowing platforms to provide personalized suggestions without compromising user privacy. Discuss real-world examples where FL is enhancing the user experience in applications like streaming services and e-commerce platforms.
Healthcare and Medical Research
Explore the application of Federated Learning in healthcare, where preserving patient privacy is paramount. Discuss how FL facilitates collaborative research across institutions by enabling model training on decentralized patient data, leading to advancements in medical diagnostics and treatment.
Challenges and Solutions in Federated Learning
Privacy Preservation
Address the challenges and solutions associated with privacy in Federated Learning. Discuss techniques such as secure aggregation, differential privacy, and homomorphic encryption that safeguard sensitive information during the model training process.
Communication Overhead
Examine the communication challenges inherent in Federated Learning, especially in scenarios with large numbers of participating devices. Discuss optimization techniques, compression algorithms, and other strategies employed to reduce communication overhead and enhance efficiency.
Federated Learning vs. Centralized Learning
Privacy and Security
Compare the privacy and security aspects of Federated Learning with traditional centralized learning models. Highlight the advantages of FL in protecting sensitive data and reducing the risk of data breaches.
Scalability and Efficiency
Discuss the scalability and efficiency gains offered by Federated Learning, particularly in scenarios where the data is distributed across a vast network of devices. Explore how FL addresses the limitations of centralized models in handling large and diverse datasets.
Federated Learning in Edge Computing
Edge Devices and IoT
Explore the synergy between Federated Learning and Edge Computing, emphasizing the role of edge devices and the Internet of Things (IoT). Discuss how FL enables intelligent decision-making at the edge while preserving data privacy.
Edge-to-Cloud Collaboration
Examine how Federated Learning bridges the gap between edge and cloud computing. Discuss the collaborative possibilities that arise when models are trained across both edge devices and centralized cloud servers, leading to enhanced performance and adaptability.
Future Trends and Innovations
Advancements in Algorithms
Explore the cutting-edge advancements in Federated Learning algorithms. Discuss ongoing research and innovations that aim to further optimize model training, reduce communication overhead, and enhance the overall efficiency of FL.
Integration with Blockchain
Examine the potential integration of Federated Learning with blockchain technology. Discuss how blockchain can enhance the security and transparency of FL models, creating a decentralized and trustless environment for collaborative machine learning.
Conclusion
In conclusion, Federated Learning emerges as a groundbreaking paradigm that redefines the landscape of machine learning by promoting collaboration without centralized data storage. Its applications across various industries, coupled with its emphasis on privacy and security, position FL as a transformative force in the evolution of artificial intelligence. As Federated Learning continues to mature, the prospects of collaborative intelligence and decentralized machine learning pave the way for a future where innovation converges with privacy, setting new standards for intelligent and responsible data utilization.
If you want to be aware for the latest trends. Let’s visit this link; https://kongotech.org/trendzguruji-me-awareness/
Leave a Reply